In recent years, the rapid advancement of consumer-grade GPUs, exemplified by powerhouses like the NVIDIA RTX 4090, has led some to speculate whether these high-performance cards could replace the venerable data center GPUs in professional settings. While tempting, this notion overlooks the crucial distinctions that make data center GPUs irreplaceable for many mission-critical applications.
At first glance, the impressive performance of consumer GPUs might seem to make them a viable alternative to their data center counterparts. After all, a single RTX 4090 can deliver incredible processing power at a fraction of the cost of professional-grade GPUs like the NVIDIA Tesla V100. However, the true value of data center GPUs lies not just in raw performance, but in their specialized features, reliability, scalability, and comprehensive ecosystem support - attributes that are essential for enterprise, scientific, and large-scale AI applications.
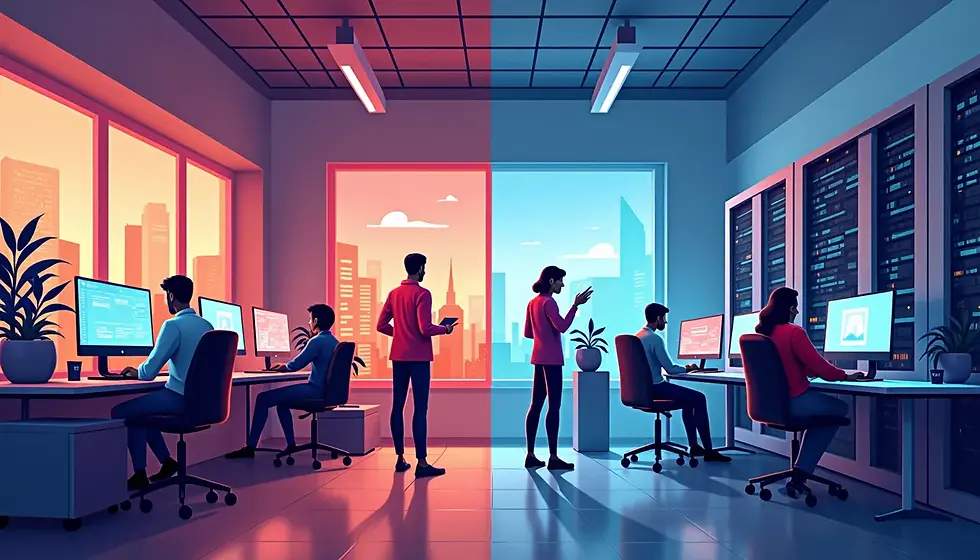
One of the primary differences lies in memory capacity. Data center GPUs often boast significantly more memory than their consumer counterparts, with the V100 offering up to 32GB of high-bandwidth HBM2 memory, compared to the 24GB of GDDR6X in the RTX 4090. This extra memory can be crucial for handling large-scale machine learning models or complex scientific simulations that require extensive data processing.
Moreover, data center GPUs are engineered to operate under sustained heavy loads, with features like ECC (Error-Correcting Code) memory to ensure data integrity, and robust power delivery systems and cooling solutions to maintain consistent performance. Consumer GPUs, while impressive, are not designed for the level of constant, intensive use required in data center environments.
Scalability is another key differentiator. Data center GPUs are optimized for use in large, multi-GPU systems and clusters, with technologies like NV Link enabling much faster GPU-to-GPU communication. This is essential for distributed computing tasks and the training of massive AI models, where the ability to efficiently harness the power of multiple GPUs is paramount.
In addition to hardware capabilities, data center GPUs also come with enterprise-grade software support, including optimized drivers and integration with professional-grade applications and AI frameworks. This level of optimization and support is crucial for ensuring seamless integration and maximum efficiency in mission-critical systems.
While consumer GPUs can certainly be capable of running many professional applications, they may not offer the same level of performance, reliability, or support as their data center counterparts. For certain scientific applications, such as those requiring high-precision FP64 calculations, data center GPUs can significantly outperform consumer cards, making them indispensable for advanced research and simulation.
Furthermore, data center GPUs offer features like GPU virtualization, remote management, and extended warranties - all of which are essential in enterprise and research environments, but largely absent in consumer-grade products.
In conclusion, while the impressive capabilities of consumer GPUs like the RTX 4090 may tempt some to consider them as replacements for data center GPUs, the reality is that these two classes of hardware serve fundamentally different needs. Data center GPUs remain irreplaceable for many professional applications, offering specialized features, reliability, scalability, and comprehensive ecosystem support that are crucial for driving innovation in enterprise, scientific, and large-scale AI domains. As GPU technology continues to evolve, the complementary roles of consumer and data center GPUs will likely persist, each serving its distinct purpose in the ever-expanding landscape of computing.
Comments