Artificial intelligence (AI) models, the brains behind the AI technology, have evolved significantly, moving from simple rule-based algorithms to sophisticated systems that can understand, learn, and even think in ways equivalent of human intelligence. These models, powered by vast amounts of data, are not just revolutionizing how we interact with technology but are reshaping industries, enhancing creativity, and opening new frontiers in research and development.
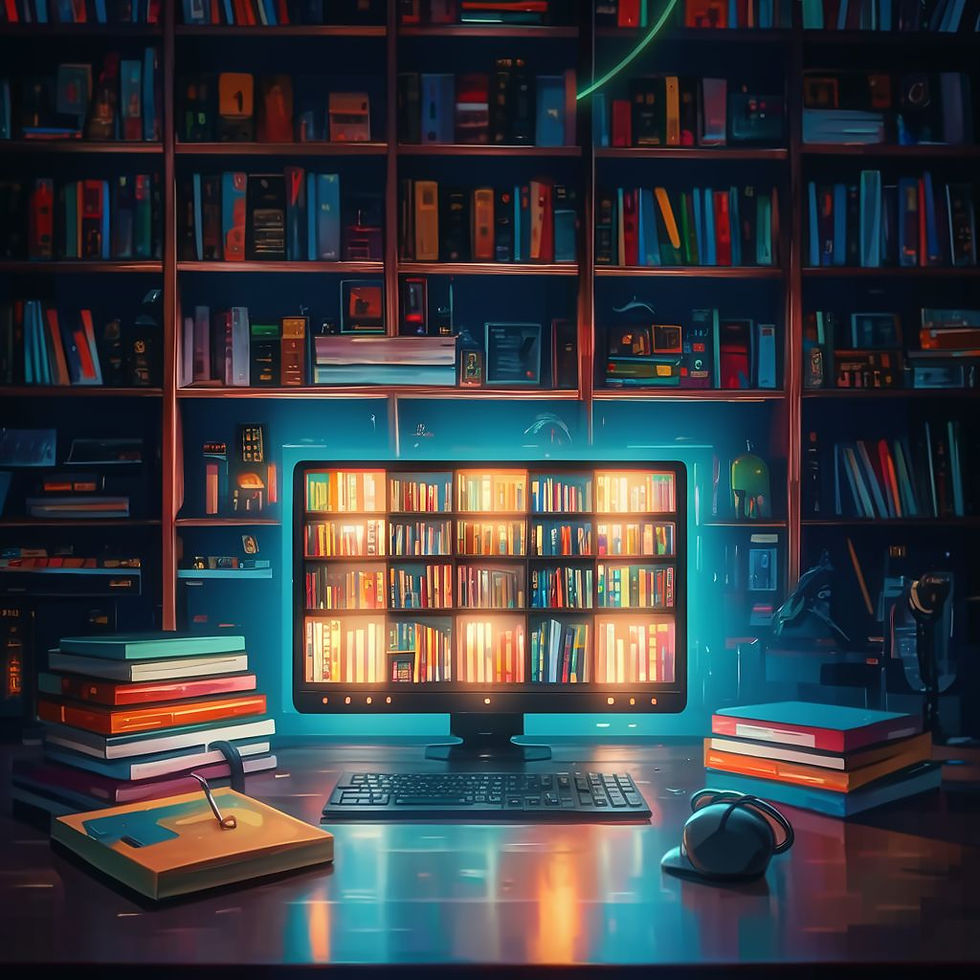
Retrieval-Augmented Generation, or RAG, is a bit like giving your computer a library card to the vast library of the internet and teaching it how to use that card wisely. Imagine every time you had a question, you could dash to the library, find the exact books you need, and get your answers in record time. That’s what RAG does for artificial intelligence (AI) models.
At its core, RAG is about enhancing AI’s knowledge and capabilities. Here’s how it works in simple terms:
1. Feeding the Model: First, you need to give the AI some basic knowledge. This is like giving someone a primer before they start researching. For example, press releases and corporate blog posts from the last two years as the starting point. This information can be collected and organized, ready to be used by the AI.
2. The Library Card: RAG then acts as the AI’s library card, allowing it to access a wide range of documents, databases, and other resources. This isn’t just limited to what was initially fed to it but can include a vast array of external sources.
3. The Smart Researcher: Once the AI has its “library card,” it can then retrieve relevant information from its vast resources whenever it’s asked a question. This is like having a super-efficient researcher inside your computer that can quickly find you the most relevant information.
The Benefits of Retrieval-Augmented Generation
Staying Up-to-Date: One of the biggest advantages of RAG is that it ensures the AI always has access to the latest information. Since the world is constantly changing, having a system that regularly updates its knowledge base is invaluable.
Better Answers: With access to more information, the AI can provide more accurate and detailed responses. This is particularly useful for businesses that need precise information for decision-making.
Versatility: RAG can be used across various fields, from customer service, where it can help answer queries with up-to-date information, to research and development, where it can provide the latest data and findings.
Overcoming Data Limitations: Traditional AI models are limited by the data they were trained on. If an AI was trained on data up to a certain point in time, it wouldn’t know about anything that happened after that point. It’s like having a textbook that never gets updated. RAG, by continuously accessing external sources, ensures that the AI can fetch and use information beyond its initial training data, keeping its knowledge base fresh and relevant.
Cost-Effective Updates: Keeping AI models up-to-date traditionally involves retraining them with new data, a process that can be both time-consuming and expensive, requiring substantial computational resources. With RAG, the need for frequent retraining is reduced because the model can access the latest information through its retrieval mechanism. This can significantly cut down on the resources needed for fine-tuning AI models, making it a cost-effective solution for maintaining cutting-edge AI applications.
In essence, RAG transforms AI models from being static repositories of information to dynamic entities that constantly learn and evolve. It’s like turning a simple calculator into a smartphone; the basic functionality is similar, but the capabilities are vastly expanded.
By utilizing the latest in AI advancements, RAG is setting the stage for a future where AI can provide more nuanced, informed, and accurate responses, making it an invaluable tool for businesses, researchers, and anyone in need of quick and reliable information.
Comments